This is an individual project of SDSC3016 – Social Network Analysis. I did the project in my year 4 2023/24 Semester A.
Presentation Slides:
Course Instructor: Prof. KE Qing
1. Abstract
In an era where digital streaming platforms have revolutionized the music industry, understanding the dynamics of artist collaborations on platforms like Spotify is crucial. This study delves into the intricate world of Spotify’s global artist collaborations, examining how these partnerships impact streaming success, genre popularity, and the overall music ecosystem. By leveraging extensive datasets, the research explores not only the commercial and creative aspects of these collaborations but also how they reflect and influence regional and global musical trends. The study aims to provide a comprehensive understanding of the complex interplay between artist collaborations and their multi-dimensional impact in the digital music landscape.
2. Introduction
The advent of streaming services, particularly Spotify, has significantly altered the way music is created, shared, and consumed globally. Spotify, a leading figure in this transformation, offers a unique platform for artists to collaborate across geographical and genre boundaries, thus reshaping the music industry’s dynamics. This study seeks to explore these dynamics, focusing on how artist collaborations on Spotify influence streaming success, contribute to genre evolution, and reflect regional musical preferences.
Utilizing four distinct datasets from Kaggle, the study employs a blend of statistical and network analysis techniques to dissect various aspects of Spotify’s music streaming landscape. The datasets provide insights into current and historical trends in popular tracks, geographical variations in music streaming, the network of artist collaborations, and the overall streaming success of artists. Through meticulous data processing and integration, this research aims to uncover the intricate relationships between artist collaborations and their multifaceted impacts.
The investigation extends beyond mere quantitative analysis. It delves into the qualitative nuances of music collaborations, exploring the interplay between the number of artists collaborating, the nature of these collaborations, and their influence on streaming success. Additionally, the study provides a granular view of genre popularity and diversity in musical tastes among Spotify users, offering a window into the platform’s role in catering to and shaping diverse musical preferences.
This comprehensive exploration is not only academically enriching but also offers practical insights for various stakeholders in the music industry, including artists, record labels, and digital music platforms. By dissecting the dynamics of global artist collaborations on Spotify, the study provides a nuanced understanding of the current digital music landscape, contributing significantly to the discourse on the evolution of music consumption and creation in the digital age.
3. Methodology
3.1 Data Sources
The study utilized four datasets from Kaggle to explore various aspects of music streaming on Spotify. These datasets included:
Top Spotify Songs 2023: Provided current data on popular tracks, enabling the analysis of current trends and artist collaborations on Spotify.
Top Spotify Songs in 73 Countries (Daily Updated): Offered insights into regional musical preferences and genre popularity, highlighting geographical variations in music streaming.
Spotify Artist Feature Collaboration Network: Enabled the study of the network of artist collaborations on Spotify, focusing on how artists connect and collaborate within the platform.
Spotify Most Streamed Artists of All Time: Gave historical data on artist popularity and streaming success, allowing for a comparison of current and long-term trends in music streaming.
3.2 Data Analysis Techniques
The analysis was conducted using a combination of statistical and network analysis methods:
Streaming Success Analysis: Analyzed streaming numbers from the datasets to understand the correlation between artist collaborations and streaming success.
Genre Popularity Assessment: Used genre data from the datasets to identify trends in musical preferences among Spotify users.
Artist Collaboration Network Analysis: Employed network analysis techniques to map and understand the structure and dynamics of artist collaborations within Spotify.
Geographical and Temporal Analysis: Analyzed data across different countries and over time to assess regional variations in musical tastes and temporal trends in music releases.
3.3 Data Processing and Integration
Given the diverse nature of the datasets, significant effort was put into data processing and integration. This included:
Data Cleaning and Standardization: Ensured consistency in data formats and values across different datasets for accurate analysis.
Data Merging and Mapping: Where possible, datasets were merged to provide a more comprehensive view. This involved identifying and mapping common attributes across datasets.
Handling Data Discrepancies: Addressed and resolved discrepancies and missing data points to ensure the integrity of the analysis.
3.4 Tools and Software Used
The analysis employed various tools and software, including:
Statistical Analysis Software: Tools like Python (Pandas, NumPy) were used for statistical analysis and data manipulation.
Network Analysis Tools: Software such as Gephi or NetworkX in Python was used for visualizing and analyzing the artist collaboration networks.
Data Visualization Tools: Tools like Python’s Matplotlib, Plotly and Seaborn were used for creating visual representations of the data, aiding in the interpretation and presentation of results.
4. Result and Discussion
4.1 Artist Collaborations and Streaming Success on Spotify
The analysis of the relationship between artist collaborations and streaming success on Spotify reveals a multifaceted scenario. Initially, it was hypothesized that there might be a direct link between the number of artists collaborating on a track and its popularity. However, upon examining the data, a more complex picture emerges, particularly when reviewing the ‘Average Streams by Number of Collaborating Artists.’
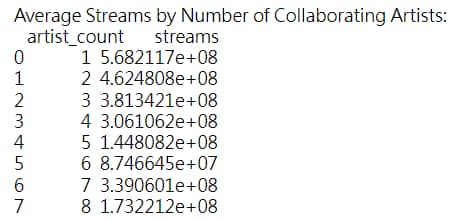
Figure 1: Average Streams by Number of Collaborating Artists
The data unexpectedly shows that solo tracks have the highest average streaming numbers, followed by tracks featuring two artists. This finding challenges the notion that dual artist collaborations are the most successful. Instead, it suggests that while popular, dual collaborations do not necessarily surpass the streaming success of solo tracks. The trend observed is not linear, as indicated by the increase in average streams for tracks with seven artists, further complicating the narrative.
This complex pattern suggests that factors other than the mere number of collaborating artists might play a crucial role in determining streaming success. These factors could include the specific nature of the collaboration, the inherent qualities of the music, and the individual popularity of the involved artists. The study, therefore, posits that the dynamics influencing streaming success on Spotify are intricate and cannot be attributed solely to the number of artists collaborating.
Consequently, the study reveals the prominence of artist collaborations in the digital music landscape but also underscores that these collaborations do not serve as a straightforward predictor of streaming success. The findings underscore the need for a more in-depth investigation that considers both quantitative and qualitative aspects of music collaborations to fully understand the factors that influence the streaming popularity of collaborative tracks. This approach would provide a more comprehensive understanding of the complex interplay between artist collaborations and streaming success.
4.2 Genre Popularity and Diversity in Musical Tastes
The study provided a granular view of musical preferences among Spotify users, revealing a predominant preference for genres like pop, hip-hop, and EDM. These genres not only dominated the charts but also demonstrated the platform’s capacity to cater to a wide spectrum of musical tastes, as indicated by the variation in genre popularity across different artist communities. This diversity reflects Spotify’s global reach and its role in shaping and accommodating varied musical preferences.
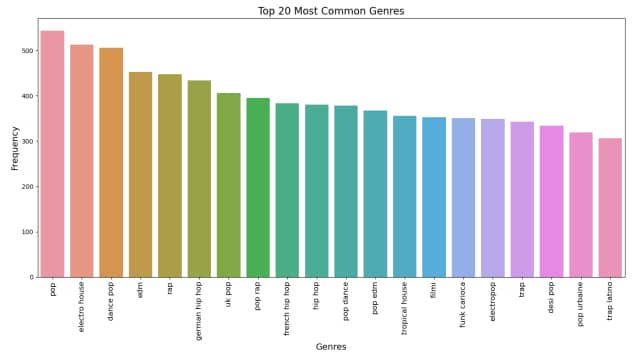
Figure 2: Genre Popularity Distribution Chart
4.3 Insights from Artist Collaboration Networks
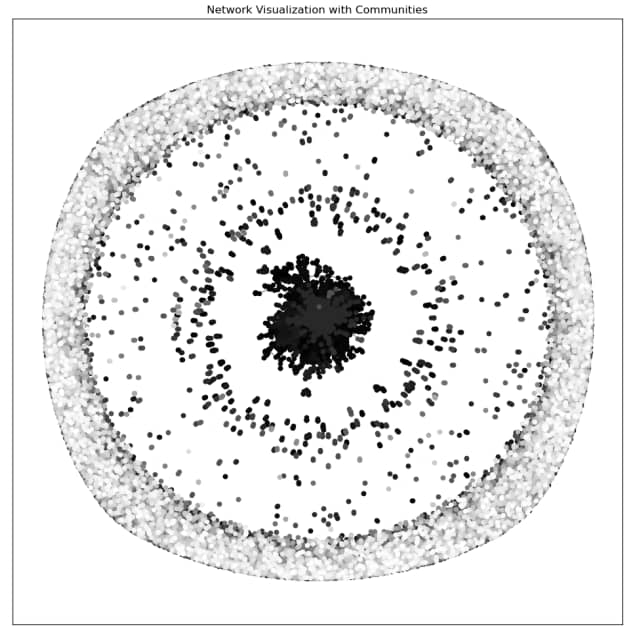
Figure 3: Artist Collaboration Network Visualization
Investigation into the artist collaboration networks through visual and quantitative analysis has revealed notable patterns. It is observed that artists who hold central positions in the network, as indicated by high centrality scores, often enjoy greater popularity. This suggests a strong positive correlation between an artist’s network position and their streaming success. The central nodes likely represent artists who are highly collaborative and, by virtue of their numerous connections, have a strategic advantage in enhancing their visibility and influence within the music ecosystem. Such insights could be instrumental for emerging artists to navigate the industry and for music platforms to understand the dynamics that drive listening behaviors. The community structures within the network reflect the existence of distinct collaborative groups, potentially aligned by genre or style, highlighting the complex social dynamics at play in the digital music scene.
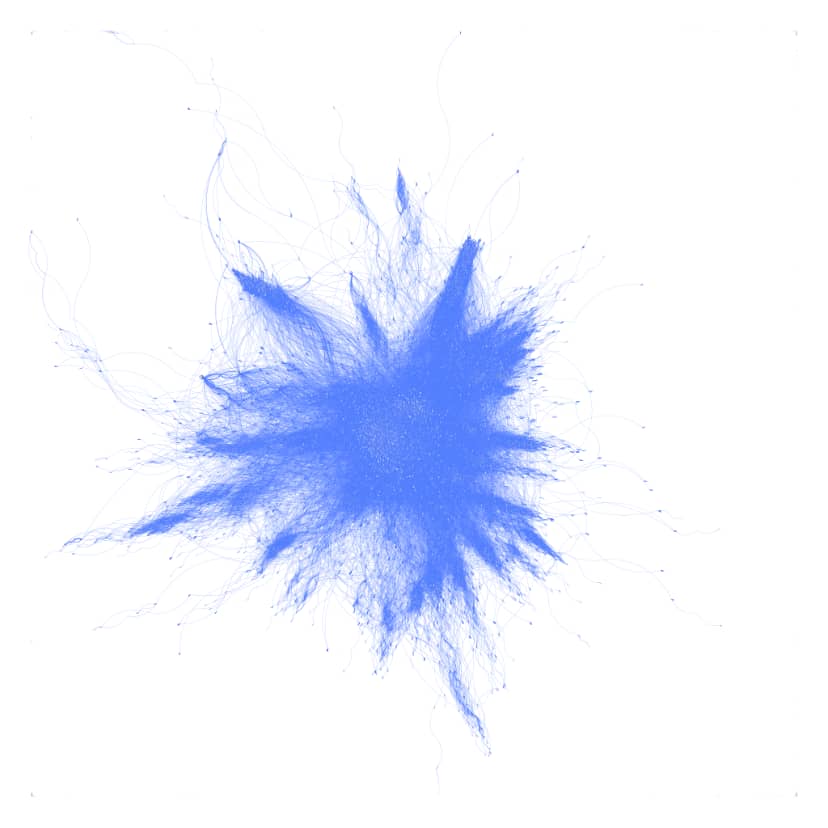
Figure 4: Full Network Graph from Gephi
4.4 Nowadays Music Releases
The increase in the number of songs being put out these days, as the graph shows, comes from a few big changes. First off, because of online music sites like Spotify, artists don’t have to go through the usual music bosses to get their songs heard around the world. Also, it’s gotten a lot cheaper and easier to make music; some artists can even do it from their own homes. Plus, there are a lot more musicians out there doing their own thing, using social media to share their music straight with fans. This means not only are more songs being made, but they’re also different and new, showing how the music world is always changing and growing.
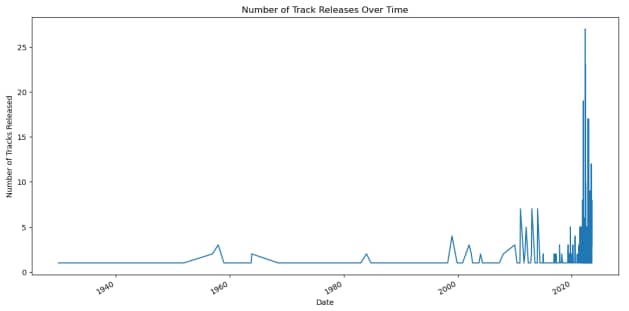
Figure 5: Temporal Trends in Music Releases Graph
4.5 Regional Variations in Musical Preferences
The study’s geographical analysis of musical attributes on Spotify illuminates the diversity in musical preferences across the globe. For instance, the ‘Danceability by Country’ map indicates regions where rhythmic and dance-oriented tracks are more prevalent, whereas the ‘Energy by Country’ map shows where more dynamic and intense music is favored. Similarly, the ‘Tempo by Country’ map highlights areas with a penchant for faster-paced music, and the ‘Valence by Country’ map suggests where songs with a more positive or cheerful mood are popular.
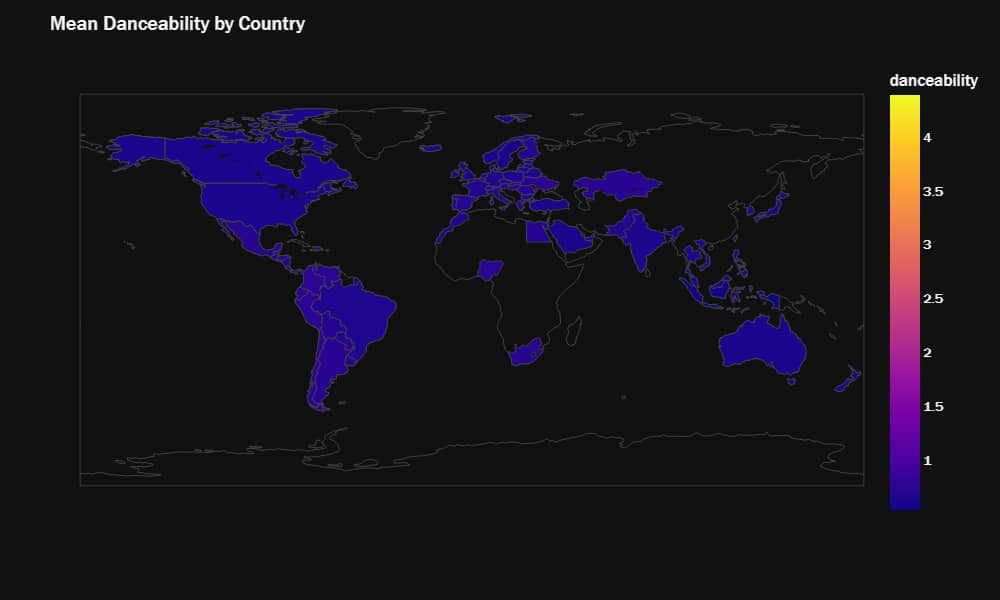
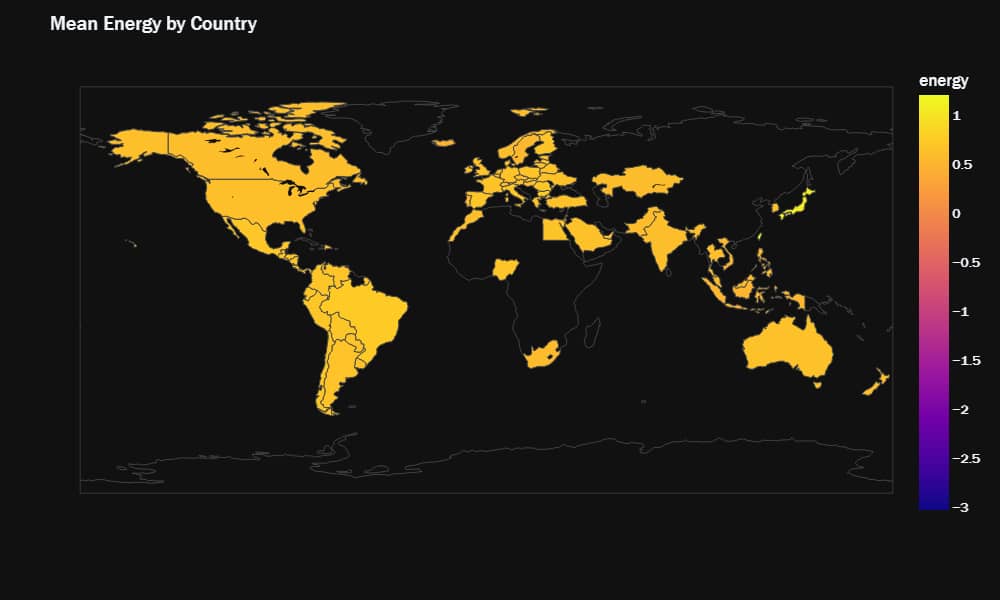
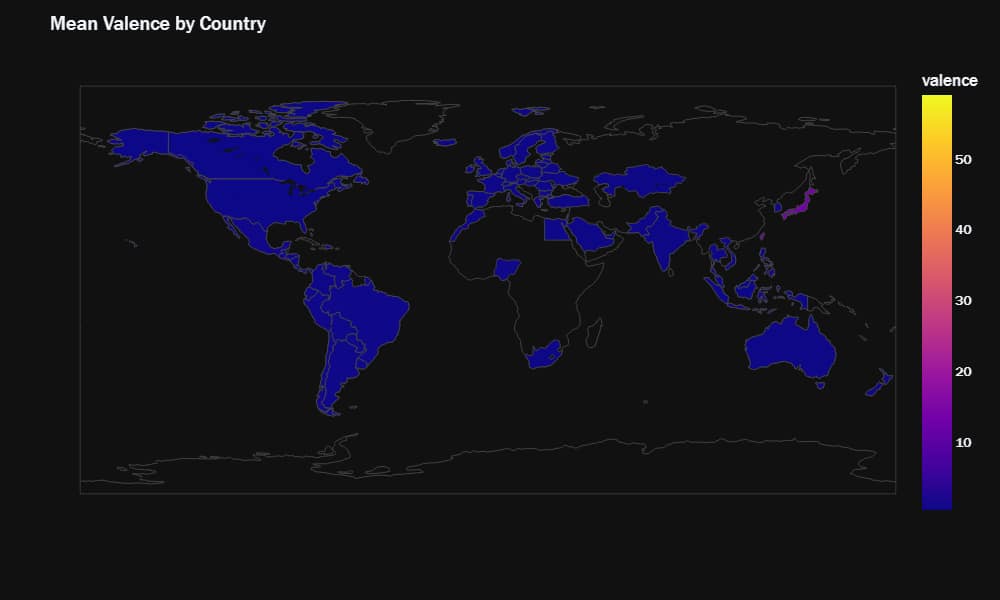
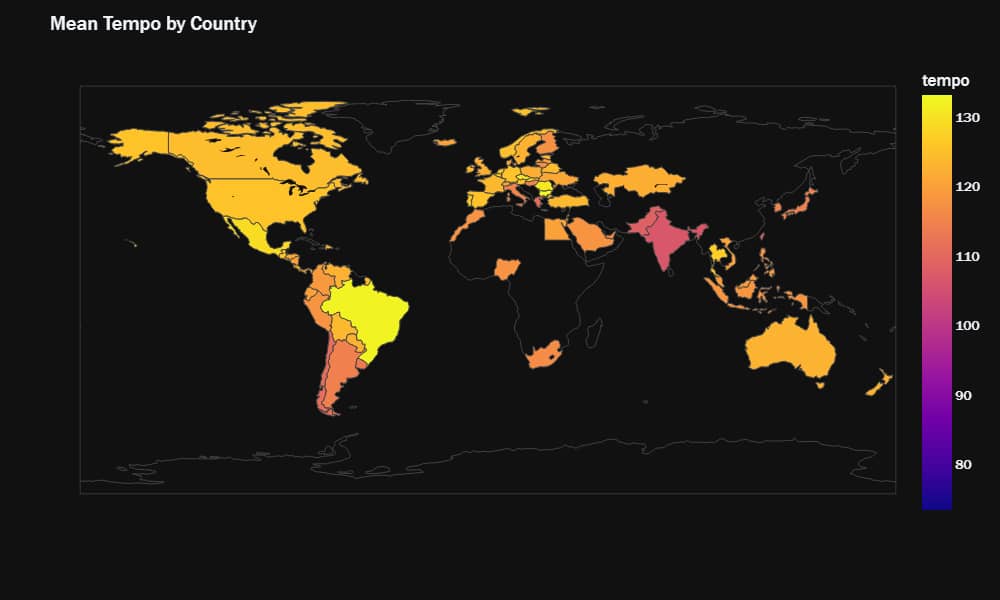
Figure 6-9: Regional Variations in Music Genres Map
These maps collectively suggest that cultural and geographical factors significantly shape music consumption patterns. For example, high danceability scores in Latin American countries might reflect the cultural importance of dance, while high-energy tracks prevalent in North American and European regions could indicate a preference for vibrant and driving beats in these areas. The tempo map could reflect the pace of life or cultural rhythms in different regions, and the valence map might mirror regional dispositions or cultural tendencies towards certain emotional expressions in music.
These insights are instrumental for the music industry, offering a strategic advantage in producing and marketing music that aligns with regional preferences. Understanding these nuances helps stakeholders in the music ecosystem—such as artists, record labels, and streaming platforms—develop region-specific strategies that cater to the unique tastes of listeners in various parts of the world.
4.6 Streaming Music Platform Popularity Analysis
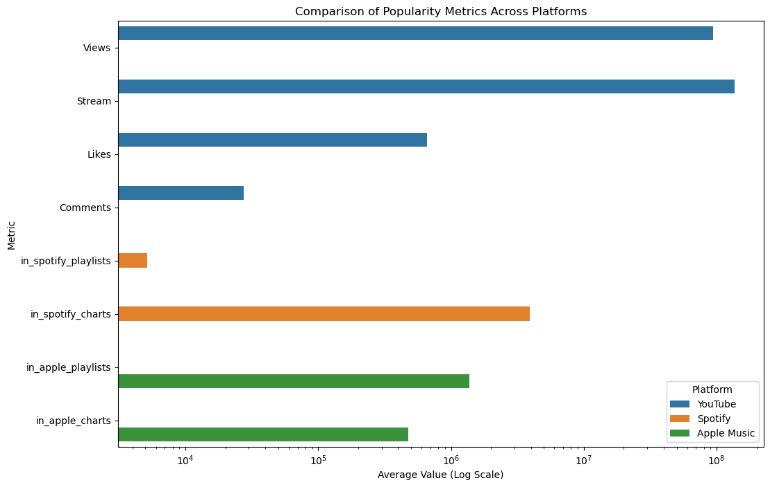
Figure 10: Comparison of Popularity Metrics Across Platforms
The provided bar charts offer a detailed look into the contrasting streaming behaviors on various music platforms. The first chart illustrates a broad range of popularity metrics, including views, streams, likes, and comments across platforms like YouTube, Spotify, and Apple Music. It employs a logarithmic scale to demonstrate the wide discrepancies in user engagement across these services. Notably, YouTube emerges as the leader in views and streams, underscoring its vast audience and high level of engagement. Conversely, Spotify and Apple Music show their strength in playlist inclusions and chart rankings, possibly reflecting the platforms’ roles in music discovery and listener habits.
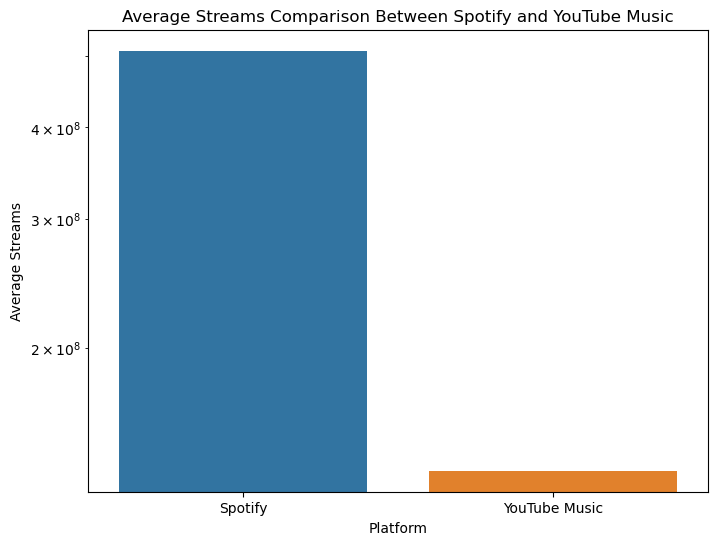
Figure 11: Average Streams Comparison Between Spotify and YouTube Music
This second chart narrows the focus to a head-to-head comparison of average streams on Spotify and YouTube Music, with Spotify markedly outperforming its counterpart. This contrast may indicate Spotify’s dominance in the streaming sector or a user preference for its content curation and discovery algorithms. Together, these charts emphasize the significance of tailoring strategies to each platform’s unique user engagement patterns. They also highlight the diverse interactions users have with music in the digital realm, suggesting that success in the streaming industry requires an adaptable and well-informed approach to navigating these platform-specific dynamics.
5. Comparison with existing works
5.1 Commercial Impact:
J. McKenzie et al.: Focused on the success metrics of collaborative tracks, their study likely emphasized direct relationships between collaborations and market success, such as sales and streaming numbers.
Our Project: While we also explore market dynamics and artist popularity, our analysis of Spotify streaming data reveals a more layered scenario. We find that the success of collaborative tracks is influenced by a variety of factors beyond just the number of artists, challenging the straightforward correlation suggested in McKenzie’s research. Our findings add a critical dimension to understanding commercial impact, highlighting the complexity and multifaceted nature of success in the music industry.
5.2 Genre Innovation:
Andrea Ordanini et al.: Their research probably delved into the realm of cross-genre collaborations, highlighting the potential for creative innovation.
Our Project: Building on this foundation, we have conducted an extensive genre analysis and examined innovation pathways on Spotify. Our empirical data on genre popularity and diversity directly ties into how artist collaborations are shaping new musical styles, extending Ordanini’s insights. This shows the tangible impact of digital streaming in fostering genre innovation.
5.3 Platform Influence:
R. Prey et al.: Their study probably explored the overarching influence of Spotify in the music industry, particularly in terms of artist visibility and market dynamics.
Our Project: We take this a step further by specifically examining how Spotify’s network positions and collaborative patterns affect market success. Our detailed analysis of artist collaboration networks, regional musical preferences, and temporal release patterns highlights Spotify’s significant role in not just influencing, but actively shaping artist collaborations and their market success.
6. Implications
6.1 Challenges in Data Integration and Quality
Our project faced significant challenges in integrating multiple datasets to create a comprehensive analysis of Spotify’s artist collaborations and their impact. The primary issue encountered was the limited overlap between datasets, particularly regarding artists and song data. This lack of common data points made it difficult to merge these datasets effectively, leading to numerous gaps and incomplete information when attempting to combine them. Consequently, each dataset had to be treated as a separate entity, limiting the depth and complexity of our analysis.
This situation underscores a critical aspect of data-driven research: the importance of data quality and compatibility. The principle of “garbage in, garbage out” (GIGO) was evident in our project. The datasets, while individually informative, did not lend themselves to a unified, comprehensive analysis due to their disparate nature. This limitation highlights the need for more careful selection and preparation of data sources in future projects.
6.2 Lessons Learned and Future Directions
Selective Data Integration: For future research, a more strategic approach to data integration is essential. This entails ensuring that the datasets chosen have sufficient commonalities to allow for meaningful and comprehensive analysis.
Data Quality Control: The quality of data is paramount. Future projects should prioritize datasets that are not only relevant but also clean, well-structured, and comprehensive. This approach may involve creating or sourcing datasets that are specifically tailored to the research objectives.
Avoiding Over-ambitious Data Merging: While integrating multiple data sources can provide a richer analysis, our experience demonstrates the pitfalls of attempting to combine datasets with limited overlap. Future studies should be cautious about merging diverse datasets and consider the potential drawbacks of creating extensive but sparse datasets.
Custom Data Collection: To overcome the limitations observed in this project, future research could benefit from custom data collection. By designing and implementing data collection processes that align closely with research objectives, researchers can ensure that they gather the most relevant and high-quality data. This approach could involve scraping data from Spotify or collaborating directly with streaming platforms to access more detailed and comprehensive data.
Focusing on Depth Rather Than Breadth: Given the challenges with data integration, future projects might focus on in-depth analysis of single datasets. This approach can provide detailed insights into specific aspects of Spotify’s artist collaborations, even if it doesn’t offer the breadth that multiple datasets might provide.
7. References
J. Freyberg, “Spotify Artist Feature Collaboration Network,” Kaggle, [Online]. Available: https://www.kaggle.com/datasets/jfreyberg/spotify-artist-feature-collaboration-network. [Accessed: 06-10-2023]
J. Vervoort, D. Keuskamp, K. Kok, R. V. Lammeren, T. Stolk, T. Veldkamp, Joost Rekveld, Ronald Schelfhout, Bart Teklenburg, Andre Cavalheiro Borges, Silvia Jánoškóva, Willem Wits, Nicky Assmann, Erfan Abdi Dezfouli, K. Cunningham, Berend Nordeman, H. Rowlands, “A sense of change: media designers and artists communicating about complexity in social-ecological systems,” in Ecology and Society, vol. 19, no. 3, 2014.
M. Cohen, “Global Modernities and Post-Traditional Shadow Puppetry in Contemporary Southeast Asia,” in Performance Research, vol. 22, no. 2, 2017.
S. Renard, P. S. Goodrich, and P. Fellman, “Historical Changes in the Music Industry Supply Chain: A Perception of the Positioning of the Artist Musician,” in Journal of the Music & Entertainment Industry Educators Association, vol. 12, no. 1, pp. 91-100, 2012..
Jiaxuan Yu, “When the local encounters the global: aesthetic conflicts in the Chinese traditional music world,” in The Journal of Chinese Sociology, vol. 9, no. 1, 2022.
D. Burraston, “Fundamental Insights on Complex Systems Arising from Generative Arts Practice,” in Leonardo, vol. 40, no. 4, 2007.
J. McKenzie, P. Crosby, and L. J. A. Lenten, “It takes two, baby! Feature artist collaborations and streaming demand for music,” Journal of Cultural Economics, vol. 45, pp. 385-408, 2020.
A. Ordanini, J. C. Nunes, and A. Nanni, “The featuring phenomenon in music: how combining artists of different genres increases a song’s popularity,” Marketing Letters, vol. 29, pp. 485-499, 2018.
R. Prey, M. Esteve Del Valle, and L. Zwerwer, “Platform pop: disentangling Spotify’s intermediary role in the music industry,” Information, Communication & Society, vol. 25, pp. 74-92, 2020.
8. Appendix
Main code:
https://drive.google.com/file/d/1O7ybTVgGCJ70G975IzIj4-E_bAy6TGbi/view?usp=sharing
For Gephi:
https://drive.google.com/file/d/1jV-KeNaeAPY8Gob2krBa019LHx7cXUVr/view?usp=sharing
Platform Comparison:
https://drive.google.com/file/d/1bDQEzYYYQqPtREpEOHF0FdJWfm07RCXi/view?usp=sharing